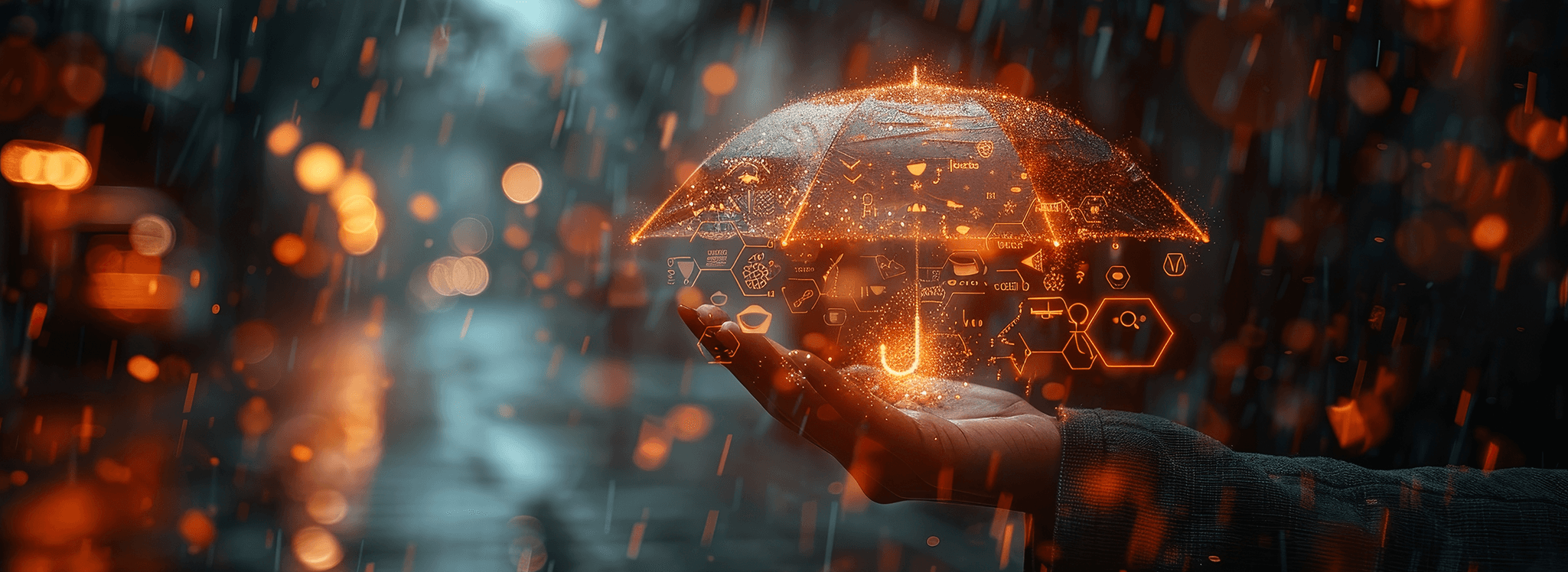
Intro
The insurance industry is quickly embracing artificial intelligence (AI), with 77% of executives in a recent Conning survey saying they’re incorporating AI into their value chain. This is a significant jump from 2023, when 61% were already using or planning to use AI in their workflows. The survey also found that 67% of C-Suite leaders are piloting large language models as part of their AI initiatives.
It’s true that AI can bring in impressive benefits like boosting efficiency, improving customer experiences, and driving innovation. For example, a study of the impact of genAI on the industry between 2021-24 shows that insurance brands are now seeing an up to 95% higher accuracy of fraud detection, and 80% faster query handling from consumers.
But what if we told you that AI isn’t always the best choice for every project? Our research at Clurgo shows that nearly 40% of proposed AI initiatives would deliver better ROI through alternative approaches.
That’s why it’s important to validate your assumptions to ensure that AI is the right fit for your company’s specific needs.
In this article, we’ll walk you through the recommended roadmap of AI insurtech projects
– from internal preparation to potential wide-scale adoption.
Step 1: Preparing for AI internally
The first step in successfully implementing AI is making sure your organization is ready for it.
What does ready mean exactly? That you should:
-
- Have an identified area you believe could perform better with the help of AI (for example, your team spends too much time on manual claims’ verification)
- Have access to data and the ability to analyze it, as data will form the core for introducing AI in insurance.
This will let you check that you have a solid foundation for AI adoption and ensure the solution integrates smoothly into your operations.
Executive insight: not every project or process requires AI – we discuss such scenarios in the next steps.
That said, we often encounter clients who approach us with little preparation. For example, one client came to us with a concept for analyzing consumer behavior at major retail chains. However, they came up with the idea only and hadn’t done any groundwork. Without that crucial preparation, unfortunately, there’s not much either side can do to move things forward.
AI isn’t just about hitting functional targets; it requires a cultural shift. You need to feel that the process is manageable and won’t require too much time or effort. If it feels too overwhelming, most companies may decide against moving forward.
From our experience, a lot of companies come with an assumption “We have all the data needed to make a decision on AI adoption”. However, when we dive in and discuss the intricacies of their data, it often turns out to be the opposite. That’s where workshops can be helpful.
At Clurgo, in just 3-5 days, we help clients assess whether they should use an external solution or build one in-house. These workshops help clarify costs, potential ROI, and give us a better sense of the client’s readiness to start a long-term partnership. It’s also a great way to set expectations and understand the client’s goals from the outset.
By the end of the workshop, we want clients to feel they have a concrete, actionable plan in place. Whether they’re focusing on improving claims processing, underwriting, or another area, the goal is for them to walk away knowing exactly how the process works and how AI can help.
At the same time, it’s important to gather insights from the client about their unique challenges. This way, we can create a tailored approach that truly works for them.
Sometimes, though, the ROI is lower than expected, and senior management may decide to pull the plug so setting the right expectations early is critical.Here’s a little tip from us on deciding if or where to implement Artificial Intelligence in insurance:
The real opportunity for AI lies in processes that involve lots of people doing low-skilled, repetitive tasks. Things like reading documents or verifying forms. These manual processes take up time and resources, and that’s where optimization really pays off. By automating these tasks, you can free up valuable resources and save hours every day.
For instance, after introducing AI in claim processing for one of our clients, 85% of claims are now auto-resolved within a day from the moment they gather all necessary documents instead of four, when they were handled manually.
The key to success is focusing on scale – the number of hours you can save by automating these labor-intensive tasks. A strategic approach to acquiring AI talent involves evaluating internal capabilities and pinpointing key skill gaps. This may require complementing in-house teams with specialized external expertise to reduce implementation risks and speed up project timelines.
Step 2: Building fundamentals internally
The next step is creating a strong foundation within the company. Whether you choose to develop AI solutions internally or work with external partners, it’s essential to remain fully engaged and invested in the process.
Many companies face the challenge of lacking the necessary expertise or having skilled professionals who are already stretched thin. So, it’s about time and expertise.
Talent is always in high demand, and there’s never enough to go around. While it’s possible to build an AI solution in-house, the question remains: what are the real chances of success?
That’s where we come in. By bringing in top-tier talent, we help bridge the gap and reduce the risks associated with internal development. Having external experts on board ensures you have a reliable backup plan, giving you peace of mind while your team focuses on other priorities.
Step 3: Validation and benchmarking workshops
Such workshops are crucial for companies exploring if AI is the right solution or just one of several options. This matters, because not all AI in insurance use cases are the same. Even more so, not all organizations have the internal capacity to assess whether AI is the go-to solution or simply an additional layer of complexity.
For example, one of our clients wanted to use AI to analyze call center conversations in the pre-LLM era. They turned to a ready-made solution and used it to record calls and convert them to text. The goal of the AI was to assess if the agents adhered to the correct communication guidelines.
However, the initial implementation struggled, so they turned to external consultants and built an internal cloud-based system, which led to much better results. In another case, the same company considered using AI to assess health impairments. AI could analyze medical documentation and determine the percentage of impairment, speeding up the process and increasing accuracy.
Tip: Before deciding on a solution, identify the most pressing challenges your company faces.
As a tech partner, we always aim to understand our clients’ pain points, because they help us guide them in who should handle the implementation and how to best allocate the budget for pilot projects.
The goal isn’t to push AI for the sake of it, but to ensure the solution is the right fit for their needs.
A well-defined validation process, incorporating detailed benchmarking and ROI analysis, is crucial for assessing the effectiveness of AI solutions. This process should consider alternative approaches and focus on ensuring alignment with strategic business goals.
The question is: how far should the pilot go? If it proves successful, can it be expanded beyond one use case, such as improving call center operations? In our experience, many successful pilots started small but eventually scaled to broader applications across the organization.
When agreeing on these pilots, it’s essential to define the collaboration process with your internal team. Some might be hesitant towards an external team taking full ownership, but it doesn’t have to be that way. A hybrid approach, where external experts work alongside internal teams, can work wonders in ensuring a smooth implementation.
Executive insight: Before approving any AI budget, require a validation assessment comparing AI to alternative approaches with projected ROI timelines for each.
Mitigating risks early on is what we always do
Risk is always a factor, but what we do differently from other tech partners is that we aim to mitigate project risks. By guiding clients through the unknowns, we minimize uncertainty which is the most costly part of any project. We outline what we need from clients, bring our expertise to the table, and make sure risks are clearly understood throughout the process.
Unlike many software houses that follow a “give us your requirements, and we’ll build it” approach, we focus on delivering results that optimize business, reduce costs, and improve efficiency. We know that, from a C-level perspective, it’s not just about building something new: it’s about ensuring the solution will deliver measurable business value.
We agree on specific metrics such as “70% of claims processing tasks automated, 30% reduction in processing time, 20% decrease in operational costs.” These figures, validated by market analysis and industry benchmarks, give executives clear, tangible benefits of implementing AI or another solution.
Finding the best way to integrate your existing systems
Another important point is customization, particularly when it comes to integrating existing systems. Many companies don’t realize that the real challenge of optimization lies not in buying and implementing off-the-shelf modules, but in handling complex integration.
Ultimately, whether AI or another solution is chosen, the primary goal remains the same: generating ROI. While savings are important, companies are ultimately interested in how they can drive revenue through technological optimization. We focus not just on reducing costs but on identifying new revenue streams for organizations.
Take one of our current clients, who is a global money transfer app, as an example. They’re not interested in purchasing new systems. They already have five reporting systems, three databases, and numerous data aggregation tools. What they want is to optimize and streamline their existing infrastructure. This is where we can help by benchmarking and refining the tools they’ve already invested in, ensuring they get the maximum value from what they already have in place.
This approach is where we make a real difference.
Step 4: Pilot of the implementation
It is essential that any collaboration with external partners is based on proactive, results-oriented cooperation, rather than passive observation. You should expect measurable ROI indicators within three to six months, as they’ll serve as a benchmark for the effectiveness of the pilot phase.
The conclusion of the pilot phase should provide practical insights that enable informed decisions about the further implementation of AI. The primary goal is to validate the feasibility of the AI solution and demonstrate its potential to deliver measurable business value.
Step 5: Wide-scale implementation and monitoring
If the pilot has been successful, the C-level team must decide on the budget and functionality for scaling the solution. The role of the AI implementation partner is to provide the company’s C-suite with output that includes a fragmentary benchmark, addressing their questions and helping them choose the best path forward (A, B, C, or D). This is crucial because the next step depends on this decision, shaping the company’s future course.
The scaling process usually takes 12 to 24 months. Unlike in many development projects which are open-ended, at Clurgo we emphasize control over ROI. It’s crucial for us that you can make adjustments as needed, maximizing ROI in the shortest iterations possible. This is essential for enterprises, as lost opportunities can lead to severed ties and reluctance to repeat the same mistakes.
Ongoing monitoring and defining success
Once scaling begins, it’s key to define success through measurable metrics. This is where Clurgo’s domain expertise in insurance technology shines. We aim to avoid vendor lock-ins by empowering clients with knowledge transfer, so they feel they have control over their solution and intellectual property (IP). The complexity lies not just in AI but in the system architecture that supports it.
Companies should define their success indicators early on, as later internal pressures may lead to cutting corners or overstating savings. Success metrics could include:
-
- Reducing claim processing time
- Improving customer reviews and online feedback
- Increased sales or cross-selling due to better consultant interactions
- Improved conversion rates.
By monitoring these areas, you can objectively assess whether your investment has paid off, thus ensuring sustainable success and value generation throughout the organization.
AI in insurance will only bring ROI if you pick the right process for optimization
When deciding on an AI implementation, the single most important stage is validation. While it can bring in strong value, AI isn’t the only option for optimizing processes.
On top of all the considerations we shared in this post, we’d also like to underline that you should work with a vertical partner with experience in highly-regulated markets. This matters particularly strongly now that new compliance regulations are coming into effect on a regional, national, or even (as in the case of the US) state-level.
With years of experience delivering insurtech projects, Clurgo can be the perfect partner.
Looking to validate your idea for an AI project? Or struggling to achieve compliance with your existing systems? We can help.